Project Description
Current Energy Earth System Models (ESM) representation of soil organic matter (SOM) and its response to future environmental changes are not certain. The objective of SOM working group is to enhance our predictive understanding of the response of SOM under future environmental changes. The team hopes to achieve our overall objective by focusing primarily on the following specific objectives:
(1) Developing model benchmarks using existing soil carbon observations and environmental controllers data that can be applied to existing models and sub-models which represent SOM dynamics.
(2) Synthesizing existing field observation and literature data to develop quantitative relationships for next-generation model structure and parameterizations.
(3) Identifying key soil processes which govern SOM dynamics at large spatial scales but are not represented in existing model structures.
(4) Quantifying uncertainties in field soil carbon observations.
Sandia researchers linked to work
- Umakant Mishra
- Sagar Gautam
- Chang Gyo Jung
In addition to the Sandian’s listed above, this working group has 53 members from 42 institutes globally.
Sponsored by
DOE-BER-EESM-RGMA program area supported by RUBISCO SFA project
Publications
- Gautam, S., U. Mishra, R. Ghimire, and C. Scown. 2023. Increased drought and extreme events over continental United States under high emission scenario, Scientific Reports, 13, 21503, doi:10.1038/s41598-023-48650-z.
- Lin, Z., Y. Dai, U. Mishra, G. Wang, W. Shangguan, W. Zhang, and Z. Qin. 2023. Global and regional soil organic carbon estimates: magnitude and uncertainties, Pedosphere, doi: 10.1016/j.pedsph.2023.06.005.
- Qin, Z., Y. Zhu, J. G. Canadell, M. Chen, T. Li, U. Mishra, W. Yuan. 2024. Carbon emissions from land use change over the past six decades, One Earth, doi:10.1016/j.oneear.2024.04.002.
- Shi, Z., F.M. Hoffman, M. Xu, U. Mishra, S.D. Allison, J. Zhou, J.T. Randerson. 2024. Global-scale convergence obscures inconsistencies in soil carbon change predicted by Earth system models, AGU Advances, 5, e2023AV001068, doi:10.1029/2023AV001068.
- Tao, F., Y. Huang, B. A. Hungate, S. Manzoni, S. D. Frey, M. W. I. Schmidt, M. Reichstein, N. Carvalhais, P. Ciais, L. Jiang, J. Lehmann, U. Mishra, G. Hugelius, T. D. Hocking, Y.-P. Wang, B. Z. Houlton, X. Lu, Z. Shi, K. Viatkin, R. Vargas, Y. Yigini, C. Omuto, A. A. Malik, G. Peralta, R. Cuevas-Corona, L. E. Di Paolo, I. Luotto, C. Liao, Y.-S. Liang, V. S. Saynes, X. Huang, and Y. Luo. 2023. Microbial carbon use efficiency promotes global soil carbon storage, Nature, 618, 981-985, doi:10.1038/s41586-023-06042-3.
- Vitharana, U.W.A., N. J. Casson, D. Kumaragamage, U. Mishra, K. Friesen-Hughes. 2024. Factors controlling the spatial heterogeneity of soil organic carbon concentrations and stocks in a boreal forest, Geoderma Regional, e00749, doi:10.1016/j.geodrs.2023.e00749.
- Wang, M., X. Guo, S. Zhang, L. Xiao, U. Mishra, Y. Yang, B. Zhu, G. Wang, X. Mao, T. Qin, T. Jiang, Z. Shi, and Z. Luo. 2022. Global soil profiles indicate depth-dependent soil carbon losses under a warmer climate, Nature Communications, 13, 5514, doi:10.1038/s41467-022-33278-w.
- Wang, Z., D. Sihi, U. Mishra, K. Todd-Brown, J. Kumar. 2024. Upscaling soil organic carbon measurements at the continental scale by multivariate clustering analysis, JGR-Biogeosciences, 128, e2023JG007702, doi:10.1029/2023JG007702.
Photos
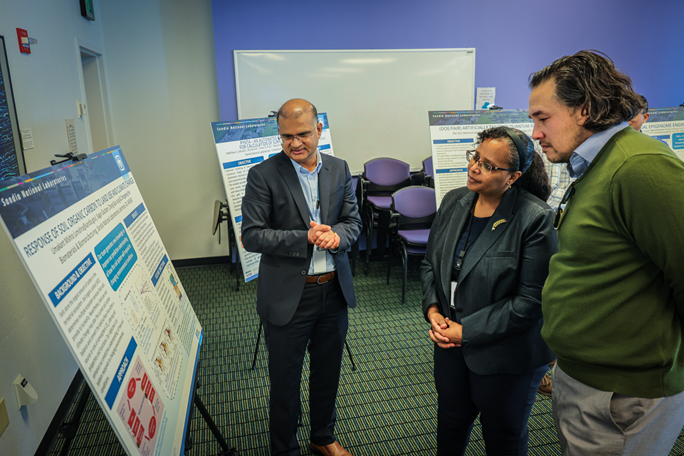
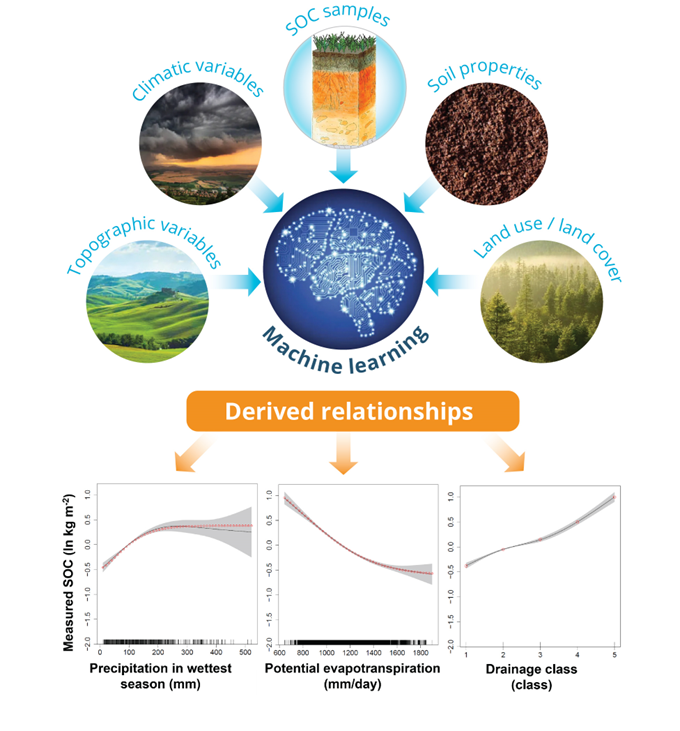
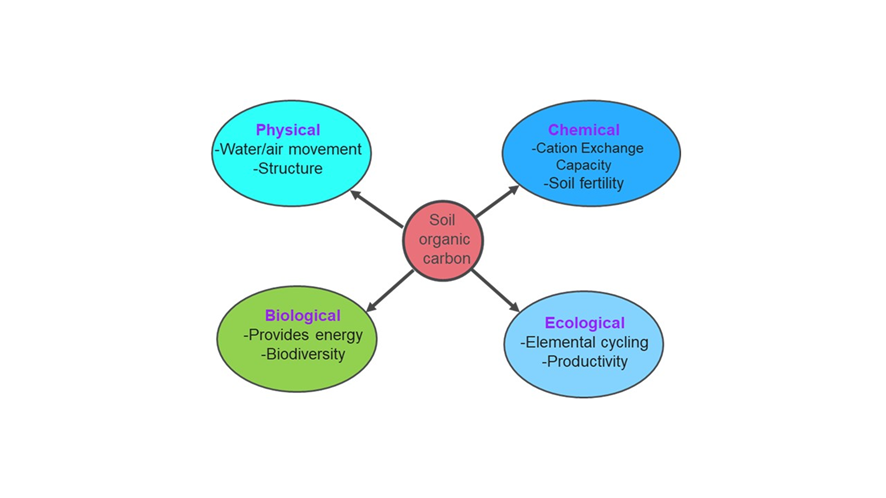
April 30, 2024