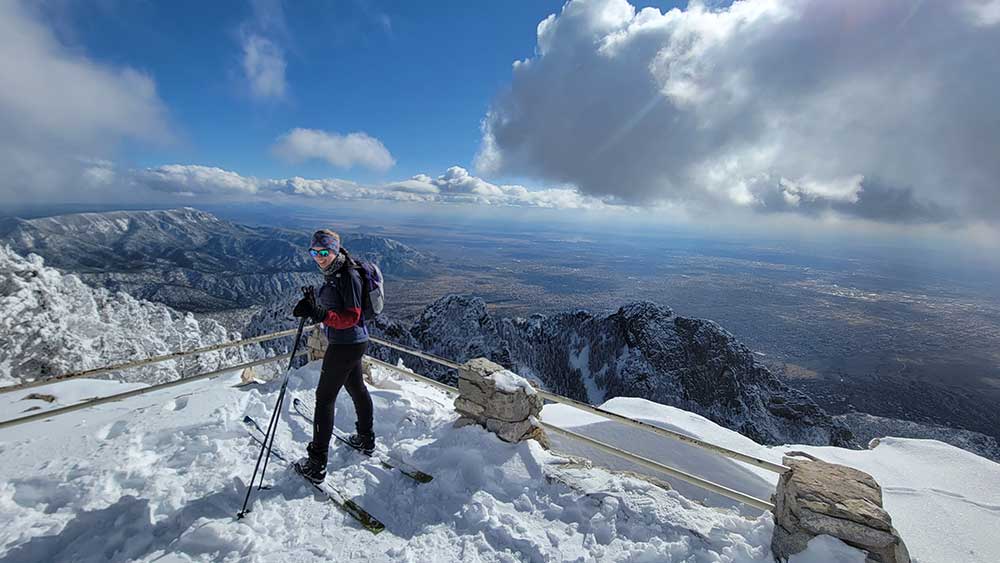
Climate change is a confusing maze of inputs and outputs. Katherine Goode spends her days trying make the variable chaos behave.
Katherine is a research and development statistician at Sandia currently supporting the CLDERA Grand Challenge. She is part of a large research team working to understand climate change mitigation strategies and their potential downstream effects. Katherine lends her statistical expertise to create novel algorithms that identify patterns and relation points in inputs and variables that climate scientists believe are related.
“I’m working with observational data collected by satellites to try to understand how different climate variables are related to some sort of climate event. It’s not an easy problem, but it’s a really interesting challenge trying to understand those relationships,” Katherine said.
Machine-learning models relate inputs and outputs to predict future outcomes. Katherine applies machine learning to better understand the negative impacts of climate change, causes and potential outcomes of future mitigation techniques — such as climate geoengineering to slow the rate of change.
“We have this really complex climate system, but we try to understand if a climate change mitigation strategy was taken, how one variable may affect another variable which will affect another variable,” Katherine said. “One of the mitigation strategies we are studying is stratospheric aerosol injections, where aerosols would be injected into the stratosphere with the intention of deflecting the sun’s rays. We would like to understand how a change in the amount of aerosols relates to temperature changes.”
Statistical relationships
For Katherine, the quest to explain variable relationships is nothing new. She graduated with a doctorate from Iowa State University in 2021 and started as a Sandia intern in 2019. Her dissertation explored the mathematical relationships between the inputs and the predictions that come out of black-box machine-learning models.
“Machine learning models are powerful tools for making predictions, but the mathematical complexity of the algorithms makes it difficult to understand how the inputs relate to the outputs. Hence the term ‘black-box models’. I work to develop techniques that provide transparency to these models. For example, if we build a model to predict temperature given the quantity of aerosols and other climate variables, how does the model make use of the aerosols for prediction,” Katherine says.
Katherine’s passion to mitigate climate change stems from her love of nature and her desire to prevent potentially catastrophic consequences in the future.
“People are developing possible mitigation strategies for dealing with climate change that could have negative downstream consequences that we still don’t fully understand. If one nation chooses to use a mitigation strategy, it could negatively affect another nation. At Sandia, we’re well positioned with individuals with varied backgrounds to solve complicated problems like this,” Katherine said.
Making connections
Because of the complicated and complex nature of both climate change and quantitative data, Katherine doesn’t hesitate to reach out to peers and constantly reads literature on diverse application spaces for statistical techniques. While she rarely has an opportunity to discuss her work with the public, Katherine takes time at conferences and events to network with researchers and fellow statisticians.
“I go to a lot of conferences, and I think that’s a place I can really talk about the application space,” shares Katherine. “There aren’t a lot of great techniques that already exist that can just be directly applied to the type of climate data that’s being worked with — which is huge amounts of data — or that can be used to address the questions that individuals have, but that’s what makes it interesting. You get to be really creative and think about how we could go about using existing tools and adapting them to work with this particular situation.”
So, what does the world look like in the future according to Katherine?
Twenty years from now, we could have a better understanding of climate change and be able to spend more time addressing other threats to national security. We could have algorithms to explain the unexplainable. But we will still need to adapt.
“I feel like we’re going to have to continue taking what we’ve learned from our current research process and I’m sure new issues will arise that we haven’t foreseen. Climate mitigation is such a meaningful place to be doing work, but it’s still a newer area of research. It’s a new application space. It’s challenging but we’ve got to overcome the hurdles,” Katherine said.
We’re going to have to learn from the present to predict the future — even when the variables don’t always behave.