Hardware and software improvements shorten ‘run time’ from year to a day
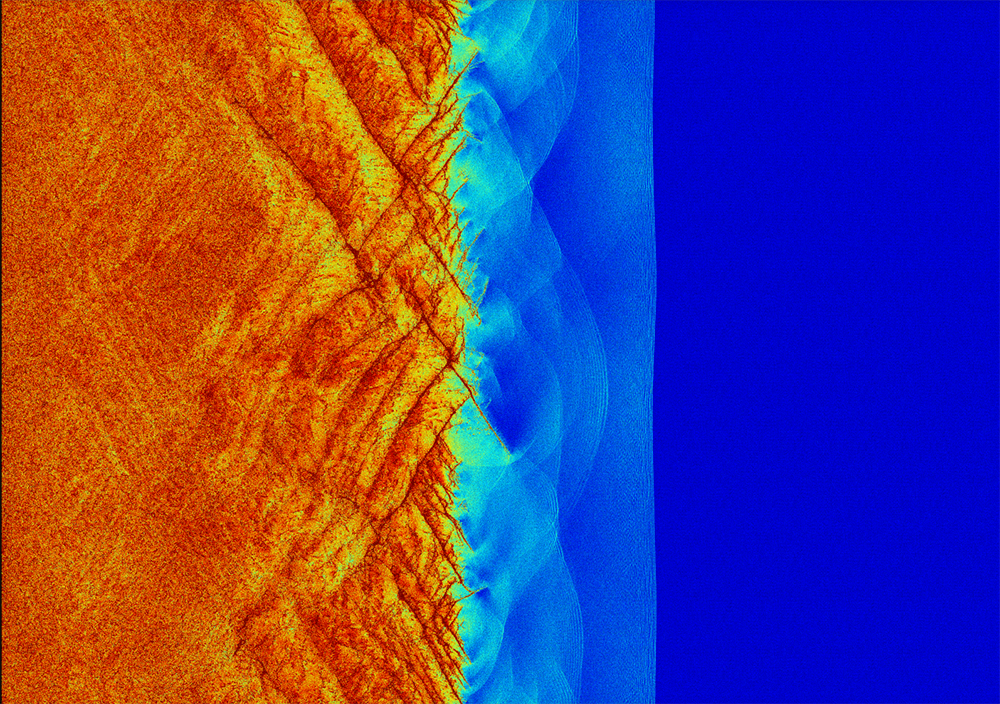
A Sandia supercomputer simulation model called SNAP, or Spectral Neighbor Analysis Potential, that rapidly predicts the behavior of billions of interacting atoms has captured the melting of diamond when compressed by extreme pressures and temperatures.
At several million atmospheres, the rigid carbon lattice of the hardest known substance on Earth is shown in SNAP simulations to crack, melt into amorphous carbon and then recrystallize. The work could aid understanding of the internal structure of carbon-based exoplanets and have important implications for nuclear fusion efforts that employ capsules made of polycrystalline diamond.
Designing novel materials and implications for giant planets
“We can now study the response of many materials under the same extreme pressures,” said Sandia scientist Aidan Thompson, who originated SNAP. “Applications include planetary science questions — for example, what kind of impact stress would have led to formation of our moon? It also opens the door to design and manufacture of novel materials at extreme conditions.”
The effect of extreme pressures and temperatures on materials also is important for devising interior models of giant planets. Powerful DOE facilities like Sandia’s Z Pulsed Power Facility and Lawrence Livermore National Laboratory’s National Ignition Facility can recreate near-identical conditions of these worlds in experiments that offer close-up examinations of radically compressed materials. But even these uniquely powerful machines cannot pinpoint key microscopic mechanisms of change under these extreme conditions, due to limitations in diagnostics at the level of atoms.
“Only computer simulations can do that,” said Aidan.
Gordon Bell finalist is about ‘a micron-sized hunk of compressed diamond’
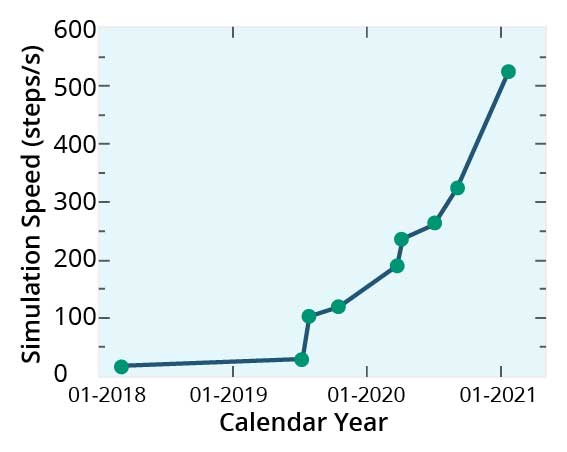
A technical paper describing the simulation was selected as a finalist for the Gordon Bell Prize, sponsored annually by the Association of Computing Machinery. The diamond-specific modeling, which took only a day on the Summit supercomputer — the fastest in the U.S. — at Oak Ridge National Laboratory, was led by professor Ivan Oleynik at the University of South Florida. In addition to Sandia and university partners, the collaborative team also included software developers at DOE’s National Energy Research Scientific Computing Center and Nvidia Corp.
The team’s simulations relied on SNAP, one of the leading machine-learning descriptions of interatomic interactions, to model and solve a very important problem, said Aidan. “We created gigantic simulations of a micron-sized hunk of compressed diamond. To do this, we track the motion of billions of atoms by repeatedly calculating the atomic forces over very many, exceedingly tiny, intervals of time.”
Machine learning bridged with quantum mechanical calculations
SNAP used machine learning and other data science techniques to train a surrogate model that faithfully reproduced the correct atomic forces. These were calculated using high-accuracy quantum mechanical calculations, which are only possible for systems containing a few hundred atoms. The surrogate model was then scaled up to predict forces and accelerations for systems containing billions of atoms. All local atomic structures that emerged in the large-scale simulations were well-represented in the small-scale training data, a necessary condition for accuracy.
Another critical part of the result was performance optimization of the software to run efficiently on GPU-based supercomputers like Summit, said Aidan. “Since 2018, just by improving the software, we have been able to make the SNAP code over 30 times faster, shortening the time for these kinds of simulations by 97 percent. At the same time, each generation of hardware is more powerful than the last. As a result, calculations that might have until recently taken an entire year can now be run in a day on Summit.”
Run time shortened by 97 percent
“Since supercomputer time is expensive and highly competitive,” said Aidan, “each shortening of SNAP’s run time saves money and increases the usefulness of the model.”
Sandia researchers Stan Moore and Mitchell Wood made important contributions to the SNAP model and the dramatic performance improvements.
The first version of SNAP was created in 2012 with support from Sandia’s Laboratory Directed Research and Development program. Software improvement has been supported continuously since 2017 by the DOE Exascale Computing Project, a collaborative effort of the DOE Office of Science and NNSA.