Research SLAM is a technical elevator speech on steroids
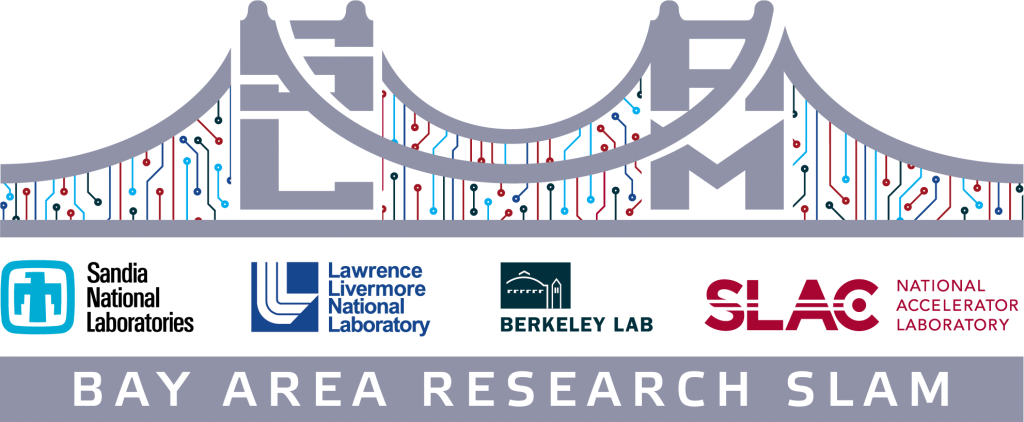
Twelve postdoctoral researchers from national labs took the virtual stage on Oct. 28 to compete in the inaugural Bay Area Research SLAM. Former Secretary of Homeland Security Janet Napolitano served as master of ceremonies for the competition, which was judged by executives from Sandia, Lawrence Berkeley National Laboratory, Lawrence Livermore National Laboratory and SLAC National Accelerator Laboratory.
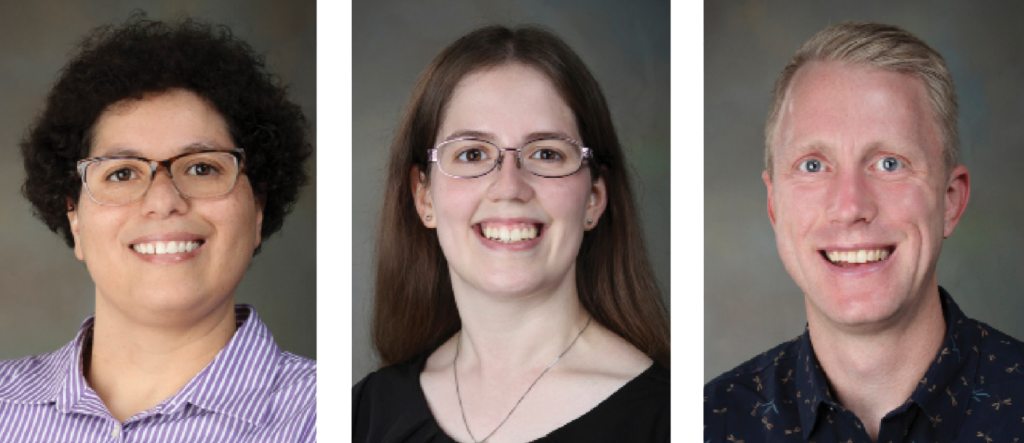
No props, the use of only one static background slide and a strict time limit made the spoken-word competition really challenging, said Sandia’s three participants.
“Three minutes and a static background are tremendous constraints that really forced me to think critically about the story being told,” said Nicole Jackson, who works in energy water systems integration.
Josh Rackers from the computational multiscale department added, “Day to day, we get wrapped up in details, but this allowed me to distill down what’s most important in my research.”
Jennifer Loe in scalable algorithms concurred. “Squeezing the main ideas into three minutes proved far more difficult than I expected. It also pushed me to explain my research with analogies for a nontechnical audience.”
A Don Quixote-style quest to simulate biological molecules with physics
Josh is on a mission.
“Since my first physics class, I’ve had a Don Quixote-style quest to use quantum mechanics simulations to help understand disease,” he said.
Companies are applying artificial intelligence to simulate biomolecules now, but no one has discovered how to piece together fragmented training data into whole simulations. Josh and his team trained a neural network to make accurate predictions on larger sets of water molecules using a much smaller fragment through an approach fusing physics and artificial intelligence.
“Electron density is key,” he said. “If we can learn the electron density for water, we might be able to do it for whatever molecule causes the next pandemic.”
Evaluating 54,000 days of ‘medical records’ for solar energy sites
Extreme weather impacts are key to Nicole’s work on a multilab effort between Sandia, the National Renewable Energy Lab and Berkeley Lab. The team utilized machine learning to correlate data gathered from the 800 sites in Sandia’s Photovoltaic Operations and Maintenance Database.
“This presents a new way of fusing together a lot of disparate datasets — production, operations and weather — to form a more complete picture of what’s happening to performance at solar energy sites,” Nicole said. “This could provide us with the ability to improve resiliency to extreme weather and gain energy output.”
Think high-precision simulations provide the best analysis? Think again.
Even supercomputers get weighed down running high-precision simulations, so Jennifer’s team ran theirs in low, 32-bit precision while utilizing multiprecision strategies on their GMRES linear solver to take smaller, more frequent steps to refine the solution. Both the mixed-precision solver and the standard double-precision solver reached the necessary high accuracy in a similar number of steps, but the low-precision solver’s steps were much cheaper and reached the solution in less time.
“We’re adding our mixed-precision refinement solver to Sandia’s widely used Trilinos library for use by scientists everywhere,” Jennifer said.
“Events like these allow scientists to practice conveying the ‘so what’ aspect of their work in a concise, relatable manner,” said Tracie Durbin, who leads Sandia’s Postdoc Program for the Chief Research Office and helped organize the Bay Area Research SLAM. “This type of critical exercise helps teach how to cast a vision of where Sandia’s research could take the nation.”
Lydia Rachbauer from Berkeley Lab won both the first place and the People’s Choice awards for her presentation “From Waste to Wanted — The Seaweed Story.”