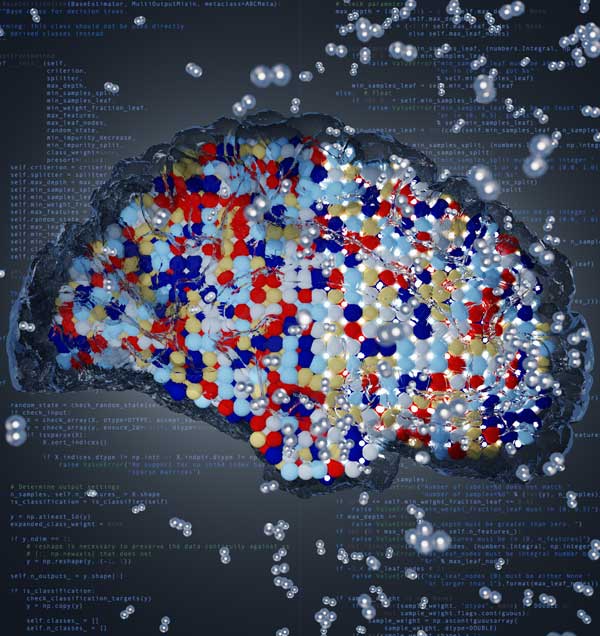
A Sandia team of materials scientists and computer scientists, with some international collaborators, have spent more than a year creating 12 new alloys — and modeling hundreds more — that demonstrate how machine learning can help accelerate the future of hydrogen energy.
Vitalie Stavila, Mark Allendorf, Matthew Witman, Jeffery Chames, Sapan Agarwal and Justin Wong — teaming with researchers from Ångström Laboratory in Sweden and Nottingham University in the United Kingdom — recently published a paper detailing their approach as the cover feature of the American Chemical Society’s “Chemistry of Materials.”
“There is a rich history in hydrogen storage research and a database of thermodynamic values describing hydrogen interactions with different materials,” Matt said. “With that existing database, an assortment of machine-learning and other computational tools, and state-of-the art experimental capabilities, we assembled an international collaboration group to join forces on this effort. The starting point was demonstrating that machine learning techniques could indeed model the physics and chemistry of complex phenomena which occur when hydrogen interacts with metals.”
Having a data-driven modeling capability to predict thermodynamic properties can rapidly increase the speed of research. In fact, once constructed and trained, such machine learning models only take seconds to execute and can therefore rapidly screen new chemical spaces: in this case 600 materials that show promise for hydrogen storage and transmission.
“Machine learning, coupled with targeted synthesis, lead to a dramatic reduction in the amount of time required to develop a new material,” Mark added. “This was accomplished in only 18 months. Without the machine learning it could have taken several years. That’s big when you consider that historically it takes something like 20 years to take a material from lab discovery to commercialization.”
The team also found something else in their work — results that have dramatic implications for small-scale hydrogen generation at hydrogen fuel-cell filling stations.
“These high-entropy alloy hydrides could enable a natural cascade compression of hydrogen as it moves through the different materials,” Vitalie said, adding that compressing hydrogen is traditionally done through a mechanical process.
He describes building a storage tank with multiple layers of these different alloys. As hydrogen is pumped into the tank, the first layer compresses the gas as it moves through the material. The second layer compresses it even further and so on through all of the layers of differing alloys, naturally making the hydrogen usable in motors that generate electricity.
Hydrogen produced under atmospheric conditions at sea level has a pressure of approximately 1 bar — the metric unit of pressure. For hydrogen to power a vehicle or some other engine from a fuel cell, it must be pressurized — compressed — to much higher pressure. For example, hydrogen at a fuel-cell charging station must have a pressure of 800 bars or higher so that it can be dispensed as 700-bar hydrogen into fuel-cell hydrogen vehicles.
“If hydrogen at 1 bar is pushed through a sequence of metal hydrides with varying thermodynamics, it automatically compresses as part of the migration from one material to another,” Vitalie explained. “As hydrogen moves through those layers, it gets more and more pressurized with no mechanical effort. You could theoretically pump in 1 bar of hydrogen and get 800 bars out — the pressure needed for hydrogen charging stations.”
Vitalie said that some American industries have already expressed interest. The team is still refining the model. However, since the database is already public through the DOE, the potential of the method could be limitless once it is better understood. In fact, Sapan said that using machine learning can lead to breakthroughs in a myriad of fields — including even more advances in materials science.
“The next step,” Vitalie suggested, “is to investigate whether the machine can tell us which order we should synthesize these materials to extract the best data, improve the model and increase the accuracy of the predictions? That’s really the future. Without all these pieces, this discovery would not have been possible.”