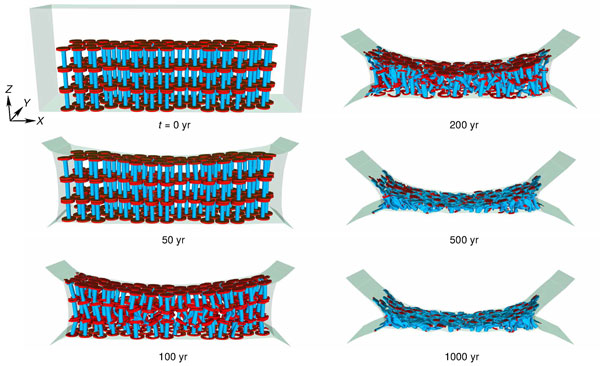
Sandia’s high-performance computing research has enhanced the understanding of exceedingly complex systems, from atmospheric flight vehicles moving through the atmosphere at hypersonic speeds, to the way waves behave 200 nautical miles off the East Coast, to the burden that the spread of COVID-19 places on medical resources across the country. These efforts and more are chronicled in the Labs’ recently released HPC Annual Report.
As detailed in the report, when COVID-19 threatened to overwhelm medical resources, Sandia high-performance computing provided critical predictive capability to inform decision-makers. It was used to model how patients with COVID-19 progressed through the hospital treatment system, as well as to predict the medical resources that would be needed to treat them, such as ICU beds, ventilators and medical personnel. The predictive work provided information essential to planning across more than 3,000 U.S. counties, including such crucial information as which hospitals would have enough of the right medical resources on hand to protect frontline workers and treat infected patients, and which states might have excess capacity to help others.
The report chronicles the Labs’ development of several new capabilities. For example, metamaterials are artificial optical structures that allow control of light in ways not offered by naturally occurring materials. They have many impressive potential applications and could even give solid material the appearance of air. Development of metamaterials has depended largely on trial and error, a problem addressed by Sandia’s R&D100 award-winning Multiscale Inverse Rapid Group-theory for Engineered-metamaterials (MIRaGE). An inverse design software, MIRaGE allows the user to start with a desired optical outcome and end with an optimized metamaterial to achieve that outcome.
Sandia high-performance computing tools brought similarly striking predictive capability to the way centuries of creeping rock salt will compress containers at the Waste Isolation Pilot Plant and how changes in design alter how much energy is lost or delivered to a target by an accelerator like the Z-Machine, while enabling better understanding of Z-Machine data.