Earth-modeling system to make high-fidelity predictions for energy
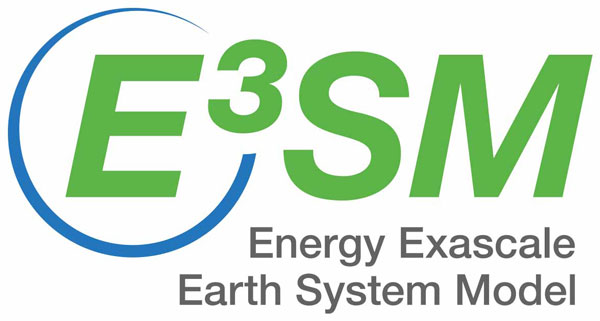
An Earth modeling system developed over the last four years and unveiled two weeks ago is expected to have one of the finest resolutions ever achieved by supercomputers simulating aspects of the planet’s climate, said Sandia researcher Mark Taylor, the project’s chief computational scientist.
The Energy Exascale Earth System Model, or E3SM, is the work of eight Department of Energy labs and several universities working under the aegis of the DOE’s Office of Science. More than 100 researchers worked to enable program users to be able to anticipate long-term changes that will critically impact the U.S. energy sector in coming years and eventually uncover other aspects of climate’s changing effects.
Simulations of the Earth’s climate, with its myriad interactions of atmosphere, oceans, land and ice components, present an extraordinarily complex system for investigation. Simulations at this grand scale must employ approximations of physical, chemical and biological governing equations on spatial grids at resolutions as fine in scale as computing resources will allow.
National labs joining Sandia in solving this problem are Lawrence Livermore, Pacific Northwest, Lawrence Berkeley, Los Alamos, Oak Ridge, Argonne and Brookhaven. The teams at these labs cover all the technical areas needed to assemble E3SM, with expertise in water-cycle science, atmosphere physics, atmosphere dynamics, aerosol modeling, land modeling, ocean and ice modeling, computational performance and software engineering.
Sandia’s work focuses on making the model run faster on today’s supercomputers and being ready to transition to the still-faster exascale computers currently under development.
“We are also making the model code easier to configure and test,” Mark said of the 30-person group he leads. “Our computer science improvements make it possible for the climate science groups to get more climate science done.”
Sandia manager Andrew Salinger leads a 10-person group working on model infrastructure, testing and development. “Sandia researchers have played vital leadership and technical roles in the development of E3SM,” Andrew said. “Our technical expertise has been critical to the development of E3SM capabilities in global atmospheric dynamics and transport, software engineering, uncertainty quantification, computational performance, land ice dynamics and unstructured-grid technologies.”
Limitations in previous computing technologies had made it impossible to develop a reliable Earth system model that would examine problems resulting from changing regional air and water temperatures, water availability and extreme water-cycle events like floods, droughts and sea-level rise. It was clear that a new model would require advances on three frontiers: increased model resolution and enhanced computational performance; more realistic rendering of the two-way interactions between human activities and natural processes, especially those affecting U.S. energy needs; and ensemble modeling to quantify uncertainty of model simulations and projections where multiple areas and factors are involved.
Technical challenges are varied. In the near term, simulating atmospheric and oceanic fluid dynamics with fine spatial resolution will be especially challenging, said Taylor. It also is important that E3SM can be effectively used by the diverse computer architectures procured by the DOE Advanced Scientific Computing Research Office.
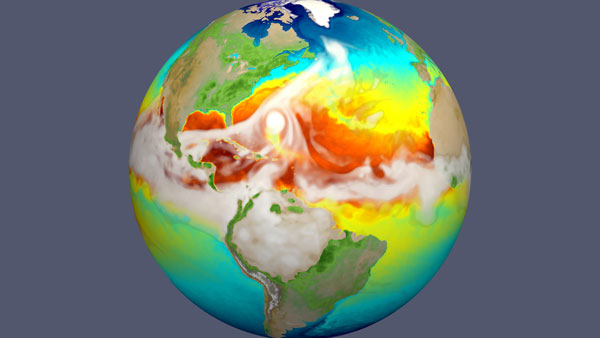
Click here to watch the model on YouTube:
A long-term aim of the E3SM project is to effectively use the exascale machines when they come online during the next five years, but since these don’t yet exist, there’s clearly a potential programming problem. But the development of E3SM is proceeding in tandem with the Exascale Computing Initiative, making it possible for each to influence the other. An exascale machine refers to a computing system capable of carrying out a billion billion (109 x 109 = 1018 ) calculations per second. This represents a thousand-fold increase in performance over that of the most advanced computers of a decade ago.
In E3SM, a big plus is that all model components (atmosphere, ocean, land, ice) can achieve variable resolution in focusing computing power on fine-scale processes in regions of interest. This is implemented using advanced mesh designs that smoothly taper the grid-scale from the coarser outer region to the more refined region.
“This model adds a much more complete representation between interactions of the energy system and the Earth system,” said Lawrence Livermore National Laboratory’s Dave Bader, the principal investigator of the E3SM project. “The increase in computing power allows us to add more detail to processes and interactions that results in more accurate and useful simulations than previous models.”
To address the diverse critical factors impacting the U.S. energy sector, E3SM strives to answer three questions:
- How does the hydrological cycle interact with the human-Earth system on local to global scales to determine water availability and water-cycle extremes?
- How do biogeochemical cycles interact with other Earth system components to influence the energy sector?
- How do rapid changes in cryosphere (continental and ocean ice) systems evolve and contribute to sea-level rise and increased coastal vulnerability?
The E3SM project benefits from DOE programmatic collaborations including the Exascale Computing Project and programs in Climate Model Development and Validation, Scientific Discovery Through Advanced Computing and Atmospheric Radiation Measurement.
Meet Sandia’s E3SM team
Sandia staff working on E3SM come from computing research, geosciences research, and reacting flow organizations. Among them are:
Jim Foucar – software engineer who works on the infrastructure used to configure, build, test and run the model
Michael Deakin – simulation workflow and provenance capturing
Erika Roesler – mesh generation, research on variable resolution capability
Wade Burgess – runs testing system
Khachik Sargsyan – land component model developing using Uncertainty Quantification methods