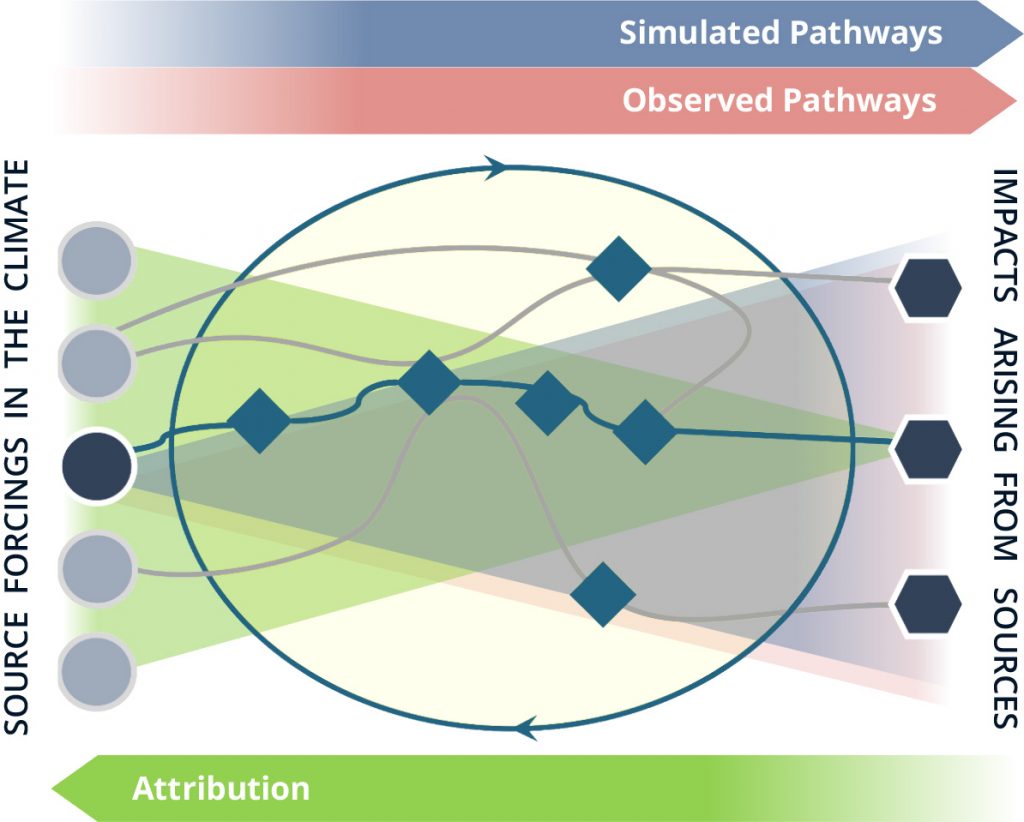
As shown in the illustration above, CLDERA combines simulations and observations to understand the physical processes that connect geographically and temporally localized climate-change sources to impacts, illustrated from left to right; resulting pathways will then be used to attribute impacts back to the source, illustrated from right to left. The goal is to transform the science of attribution to improve climate risk assessments for national security and policy decision-making. Learn more about the three research areas that make up CLDERA by clicking the buttons below.
Simulated Pathways
The aim of this thrust is to develop a fundamentally new and blended computational and information science approach to discovering and explaining connective relationships in the form of pathways between sources and impacts within E3SM simulation data and while running E3SM. We hypothesize that the discovery of simulated pathways between a source and its impacts within E3SM using a data-analytic framework will uncover etiologically sound connective relationships, rather than simply establishing piecemeal source-impact correlations. We will pursue three complementary research sub-thrusts, which fuse Sandia’s strengths in data analytics, computational modeling, and E3SM/climate science: statistical methods, computational monitoring, and anomaly detection.
Observed Pathways
The aim of this thrust is to develop novel statistical methods to uncover observationally derived pathways by accounting for dynamic spatio-temporal evolution and key atmospheric processes, multiple complementary data sources, and sources of uncertainty. We will fully exploit observational data in order to characterize the complex Earth system by developing innovative and advanced source-level data-fusion and analytical techniques so that the simulation-derived pathways may be independently substantiated. We are pursuing four complementary approaches: data fusion, change-point detection, space-time statistical methods, and hybrid statistical and machine learning (ML) methods.
Attribution
The aim of this thrust is to determine the dominant source for a given impact — it reframes the forward problem (simulated and observed pathways) to identify and rank possible sources that could have led to that impact. We hypothesize that pathways will enable attribution by overcoming natural variability to find the source signal, adding process-linked information to enable ranking of the relative contribution of a source to a specific impact, and determining source characteristics by constraining the inverse optimization problem. We are investigating three classes of complementary methods: inverse optimization using ML models, enhanced fingerprinting, and causal modeling.