Patrick Blonigan
Principal Member of Technical Staff
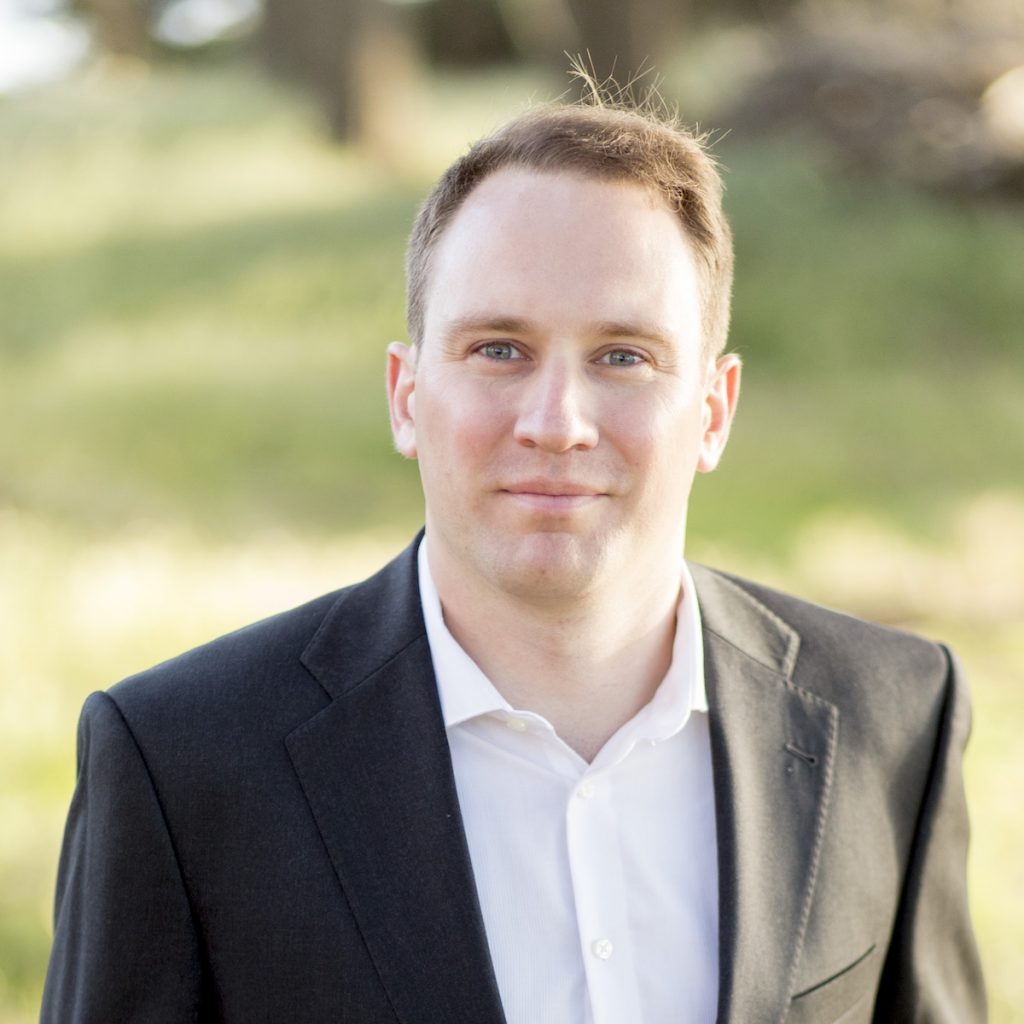
About Me
My research focuses on methods to enable engineering design and analysis with large-scale computational models. These methods include:
- Reduced order modeling. The goal of this research is to exploit any underlying structure present in data generated by a “full order” large-scale computation model to construct a “reduced order” model that is accurate, robust, and significantly less costly.
- Sensitivity analysis of chaotic dynamical systems. The goal of this research is to enable efficient design optimization, error estimation, and uncertainty quantification of large scale chaotic systems like scale-resolving turbulent flow simulations.
Although the above methods are generally applicable, my main focus is on the following application spaces:
- Unsteady Aerodynamics and Turbulence
- Hypersonics and Aerothermodynamics
Recent Publication Highlights
- Check out our paper on a compute-bound formulation of Galerkin model reduction for linear time-invariant dynamical systems. (Joint work with F. Rizzi, E. Parish, and J. Tencer)
- Check out our paper on forecasting multi-wave epidemics through Bayesian inference, with application to the COVID-19 pandemic in 2020. (Joint work with J. Ray and C. Safta.)
- Our AIAA journal paper on model reduction for hypersonic aerodynamics via conservative LSPG projection and hyper-reduction is online! (Joint work with F. Rizzi, K. Carlberg, M. Howard, and J. Fike)
News
- New paper on error estimation reduced order model in CMAME
A new paper by Eric Parish and I, titled “Evaluation of dual-weighted residual and machine learning error estimation for projection-based reduced-order models of steady partial differential equations”, has been accepted for publication by Computer Methods in Applied Mechanics and Engineering (CMAME). Read it online here.
- PRIME code
PRIME, our Bayesian framework for characterizing and forecasting partially observed epidemics, is now available on github!
To learn more visit the PRIME website or checkout this video. Additionally, my contributions to PRIME are discussed in our paper on forecasting multi-wave epidemics.
- Story on Full Airframe Sensing Technology (FAST)
Check out this story about our new collaboration with University of Texas at Austin on sensing for hypersonic vehicles.
- Pressio is now live!
Pressio, a software package we are developing at Sandia to enable projection-based model reduction for nonlinear systems, is now available on github.